How predictive analytics can help during the flu season
Predictive analytics is all about getting insights, deriving conclusions, and making grounded decisions with data. It's fast becoming a useful tool for healthcare companies and organizations to identify risks and make better business decisions
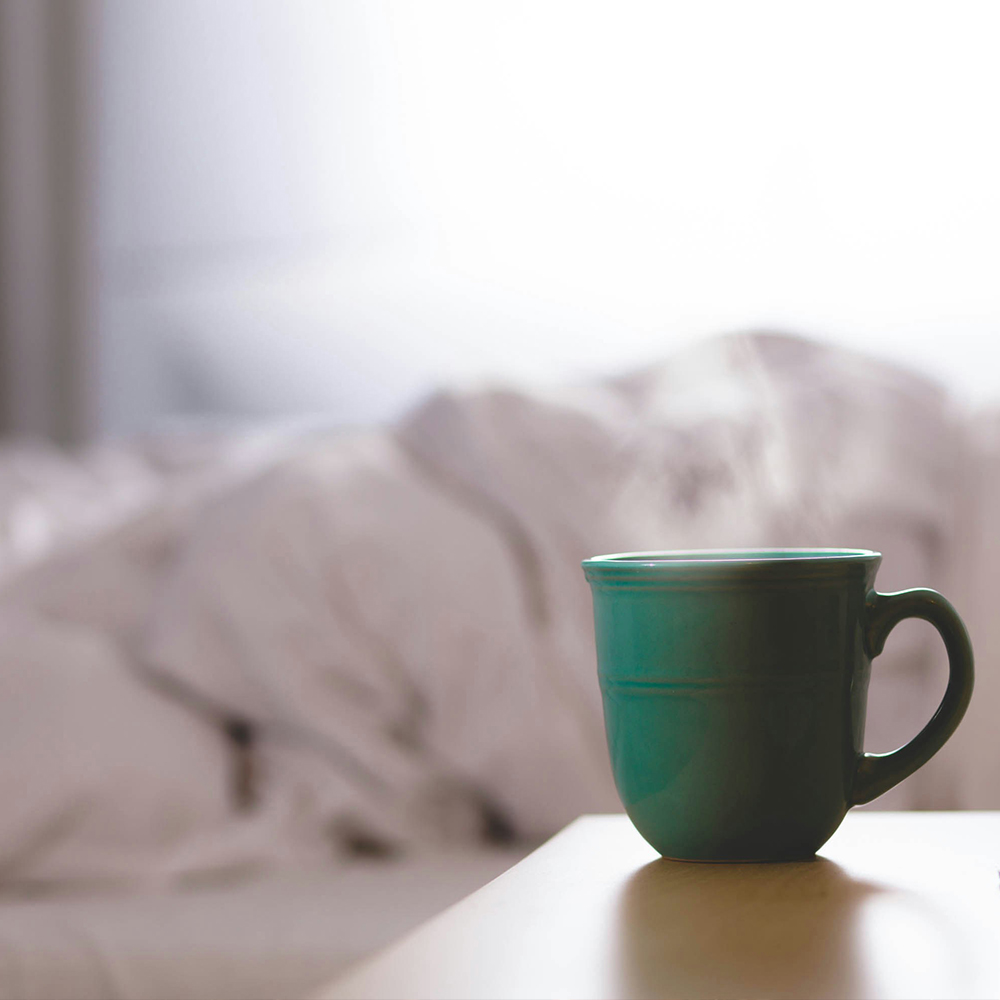
Predictive Analytics
Predictive analytics deals with the question of whether and with what probability certain events could occur in the future. For this purpose, historical data sources are used to train mathematical models that identify trends and patterns in the data. The model is then fed current data to predict the likelihood of future events.
In this way, predictive analytics is a useful tool with which companies and organizations can identify risks early on and make better business decisions. One sector that can gain much from the use of predictive analytics is the healthcare industry.
In this article, you'll learn the following:
* How predictive analytics works
* How the healthcare industry can harness its powerful potential to combat contagious diseases like COVID-19 or monkeypox
How Predictive Analytics Works
*Predictive analytics* is a term for a form of advanced analytics that can predict activity, trends, and behavior from historical data. To do this, machine learning techniques and statistical analysis are applied to data sets to create predictive models. These models can be applied to current data to identify relationships, structures, and patterns in that data, which provide inferences about possible future developments.
The predictive models give a numeric value or score for the probability that a given event will occur. This allows businesses to derive insights from data that they can use to adjust their strategy, better allocate their resources, benefit from potential opportunities, or avoid unfavorable situations.

If you're setting up a predictive analytics process for your business, below are main steps you can follow.
Project Definition
You first define the goal for your predictive analytics project; to that end, you should determine the expected results and the necessary deliverables and inputs. At this point, all the data required for the analysis should be available, up-to-date, and in a suitable format.
Data Collection
Predictive analytics uses large amounts of data to gain insights for future decisions. That's why the data collection step is crucial for the process to succeed. This will most likely involve information from multiple sources, so you'll need a unified approach to data.
The data for the predictive analytics process is usually collected in a [data lake](https://cloud.google.com/learn/what-is-a-data-lake) that contains the data in a raw format. This data can be in the form of structured tables, semistructured XML files, or unstructured social media comments.
Data Preprocessing
The data preprocessing or preparation phase includes all activities to create the final data set that's used for predictive modeling in the next step. Here, you should focus on the selection of tables, entries, and attributes, especially on the transformation and cleaning of the data. The steps involved in data preparation are as follows:
* **Data selection:** Data selection for predictive analytics strongly depends on the goals defined in the first step. At the end of this process, it should be clear which data sets are included in or excluded from the analysis.
* **Data cleansing:** A clean data set must be selected, or the data set must be cleaned to achieve the desired result during the modeling step.
* **Transformation and integration of the data:** To put the data into a usable representation form, it must first be transformed. The transformation encodes data and changes its granularity through aggregation or disaggregation.
* **Data formatting:** In some cases, a simple adjustment of the data format has to be done—for example, adjustment of the data type.
Predictive Modeling
The central step of predictive analytics is predictive modeling; this is where you actually develop models based on historical data to forecast possible future results or events. The developed model is able to generate the probabilities or value of a target variable (e.g., number of new COVID-19 cases) based on an input variable (current data).
A distinction can be made between two types of predictive models:
* **Classification models:** These can predict the probability of belonging to a certain class. For example, a classification model can predict the probability of whether a certain region or city will be a risk area for a contagious disease in the future. The probability of the model is given between 0 and 1, with 1 meaning that the scenario to be examined will occur.
* **Regression models:** These types of models predict a scalar quantity. Using the example of the COVID-19 pandemic, this variable can be a numerical value for the expected number of new COVID-19 cases in a certain region or city.
The most common predictive modeling techniques include [decision trees](https://www.ibm.com/topics/decision-trees), [linear and logistic regression](https://www.ibm.com/topics/logistic-regression), and [neural networks](https://www.ibm.com/cloud/learn/neural-networks#:~:text=Neuronale%20Netze%20spiegeln%20das%20Verhalten,und%20Deep%20Learning%20zu%20l%C3%B6sen).

Evaluation
Before the predictive model is used, it's essential you evaluate it. Does the model offer the quality to satisfy the objective of the predictive analytics project? Does the model meet the goals set at the project's beginning? If the goals are not met, it's common to iterate over the previous steps again and make adjustments and improvements until the objective is achieved.
Deployment
The deployment phase is usually the final phase of a predictive analytics project. Here, the knowledge you've gained is organized and presented in such a way that your organization can make use of it. This can include an implementation strategy, the monitoring of the validity of the models, a summary report, and a presentation.
How Can the Healthcare Industry Leverage Predictive Analytics?
Now that you've gained an overview of the predictive analytics process, we'll move on to concrete examples of how predictive analytics models offer forecasting tools that provide real-world benefits in critical situations.
Specifically, the healthcare industry can use predictive analytics to combat contagious diseases such as the flu, COVID-19 or monkeypox. Models can be used to estimate the initial spread and severity of the disease and make predictions about how the disease will develop in the future.
Predictive analytics can forecast different scenarios as a virus develops and spreads. Early information can minimize the negative impact of the virus and save lives. For instance, a predictive analytics model could predict the number of expected new COVID-19 cases or mortality rates in specific regions in the next few days. Having this information, decision-makers can calculate urgent medical needs and decide if partial or full-containment measures like lockdowns are necessary.
Furthermore, this early assessment of near-future health needs can enable better planning regarding health technologies like PPE or ventilators to ensure the required supply change and distribution. Additionally, human resources can be better managed and coordinated to ensure appropriate and quick responses.
Let's take a look at three concrete ways in which predictive analytics can help the health industry combat a disease like COVID-19.
Forecasting which Patients Would Be Most at Risk
Early in the pandemic, one of the most important use cases for predictive analytics was to determine which patients were most at risk for contracting the virus and which individuals were most likely to have a poor course of COVID-19 infection.
For this use case, a [predictive analytics classification model](https://www.thelancet.com/journals/landig/article/PIIS2589-7500(20)30217-X/fulltext#seccestitle130) was implemented using historical clinical patient data such as *age*, *preexisting conditions*, *current health status*, *minimum oxygen saturation*, etc. By applying this model to COVID-19 patients, it was possible to determine the probability of viral severity or mortality of specific patients. These findings gave hospital staff the opportunity to intervene in time and take necessary precautions to save patients' lives.
Optimizing Hospital Resources
Another issue that was prevalent in the early days of COVID-19 was the sudden increase in hospitalizations. This resulted in hospitals dealing with the challenges of limited availability of medical equipment and human resources and an additional burden on existing staff.
While hospitals are no longer in the early days of dealing with the pandemic, predictive regression models can still be extremely valuable in forecasting new localized COVID-19 cases. This information can be used to help hospitals better plan for the potential increase in patient numbers and more efficiently allocate resources. Hospital beds, ventilators, and hospital personnel can be better and more efficiently organized and prepared in advance of a surge.
Forecasting Regional Surges and Hotspots
There are few situations as unpredictable as a global health crisis. Knowing which areas will be hit hardest can help local decision-makers plan ahead against poor outcomes. Predictive analytics models can use current historical data on the spread of COVID-19 in specific regions to predict with high accuracy what will happen to that region in the coming days in terms of new COVID-19 cases.
Furthermore, historical COVID-19 data of a region can be used to predict how the virus will then spread to other regions. In this way, predictive analytics can forecast regional surges and hotspots. Having this information a few days in advance allows for implementing countermeasures in the form of social distancing rules or lockdowns.
As an additional benefit, such forecasting can be achieved using less personal data. In the early days of the pandemic, the migration of the COVID-19 virus was tracked using the travel information and contacts of people who were infected with the virus. This detailed information was difficult to obtain due to privacy concerns and availability of resources like personnel who collect, track, and evaluate this information.
Predictive analytics models do not require information on individuals' movements or contacts. These models can use data on the recent local spread of a virus from an outbreak in a certain region to forecast where, in what way, and how quickly the virus will spread in another region.
Conclusion
Predictive analytics is all about getting insights into data, deriving conclusions from it, and taking well-founded actions according to these conclusions. Ikigai Labs provides an operational BI platform that uses built-in AI-powered predictive capabilities on historical data to optimize mission-critical processes.
If you want to read insightful articles covering topics like [how to optimize purchasing decisions using sales data](https://ikigailabs.medium.com/we-know-how-much-money-you-lost-in-sales-this-year-3d1b1157c94e) or [how to automate you data operations](https://ikigailabs.medium.com/automation-of-data-operations-c3600466d3a4), you can read all about them and more on [our blog](https://ikigailabs.medium.com/).
[Ikigai Labs](https://www.ikigailabs.io/) offers an AI-augmented data processing and analytics platform that enables data business operators to build, automate, and run complex end-to-end data-driven processes. With Ikigai Labs, data operators are only a few clicks away from the following:
* Extracting structured and unstructured data from various data sources without the need to manually clean or prepare the data
* Building, managing, and monitoring several "human in the loop" automations without the need of coding them
* Using AI to receive recommendations and simulating what-if scenarios